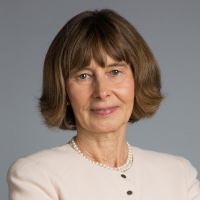
Marta Kwiatkowska
University of Oxford
Robustness Guarantees for Bayesian Neural Networks
Tue Sep 13, 2022, 9:00-10:00
Bayesian neural networks (BNNs), a family of neural networks with a probability distribution placed on their weights, have the advantage of being able to reason about uncertainty in their predictions as well as data. Their deployment in safety-critical applications demands rigorous robustness guarantees. This paper summarises recent progress in developing algorithmic methods to ensure certifiable safety and robustness guarantees for BNNs, with the view to support design automation for systems incorporating BNN components.
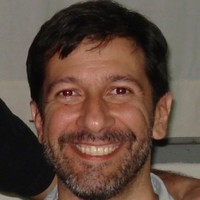
Pedro R. D'Argenio
FAMAF - Universidad Nacional de Córdoba
Analysis of Highly Reliable Repairable Fault Trees via Simulation
Wed Sep 14, 2022, 9:00-10:00
Dynamic fault trees (DFTs) are widely adopted in industry to assess the dependability of safety-critical equipment. Since many systems are too large to be studied numerically, DFTs dependability is often analyzed using Monte Carlo simulation. A bottleneck here is that many simulation samples are required in the case of rare events, e.g. in highly reliable systems where components seldom fail. Rare event simulation (RES) provides techniques to reduce the number of samples in the case of rare events. In this talk, I will present the theory that leads to the implementation of an importance splitting based toolchain to simulate a variant of repairable fault trees (RFT) whose stochastic behaviour includes non-Markovian continuous distribution. The semantics of RFT is formally defined in a compositional way in terms of input/output stochastic automata, ensuring that the resulting object is amenable to simulation. Moreover, RES requires meta-information that is usually provided by an expert. Instead, we provide a fully automatic way to derive such necessary data.